Topic modeling is a popular technique in NLP for discovering abstract topics that occur in a collection of documents.
BERTopic leverages BERT to generate contextualized document embeddings, capturing semantics better than bag-of-words. It also provides excellent topic visualization capabilities and allows fine-tuning topic representations using language models like GPT.
For this example, we use the popular 20 Newsgroups dataset which contains roughly 18000 newsgroups posts
from sklearn.datasets import fetch_20newsgroups
docs = fetch_20newsgroups(subset='all', remove=('headers', 'footers', 'quotes'))['data']
In this example, we will go through the main components of BERTopic and the steps necessary to create a strong topic model.
We start by instantiating BERTopic. We set language to english
since our documents are in the English language.
from bertopic import BERTopic
topic_model = BERTopic(language="english", verbose=True)
topics, probs = topic_model.fit_transform(docs)
After fitting our model, we can start by looking at the results. Typically, we look at the most frequent topics first as they best represent the collection of documents.
freq = topic_model.get_topic_info()
freq.head(5)
Topic | Count | Name | Representation | Representative_Docs |
---|---|---|---|---|
0 | 1823 | 0_game_team_games_he | [game, team, games, he, players, season, hockey, nhl, league, played] | [“\n\n”Deeply rooted rivalry?” Ahem, Jokerit has been in the Finnish league for two seasons.\n\n\n\n\n\nThe reason why they’re…] |
1 | 630 | 1_key_clipper_chip_encryption | [key, clipper, chip, encryption, keys, escrow, government, secure, privacy, public] | [“\nI am not an expert in the cryptography science by any means.\nHowever, I have studied the Clipper chip proposal extensively\nand have some thoughts on the matter…\n\n1) The…”] |
2 | 527 | 2_idjits_ites_cheek_dancing | [idjits, ites, cheek, dancing, yep, consistently, considered, wrt, miller, observations] | [“consistently\n\n\n, \nYep.\n, \nDancing With Reality Cheek-to-Cheek to Stay Considered Consistent and On-the-Cheek\n\nSome of Miller’s keener observations (and…”] |
3 | 446 | 3_israel_israeli_jews_arab | [israel, israeli, jews, arab, arabs, jewish, palestine, peace, land, occupied] | [“\nThis a “tried and true” method utilized by the Israelis in\norder to portray anyone who is critical of Israeli policies as\nbeing anti-Semitic…”] |
-1 | 6789 | -1_to_the_is_of | [to, the, is, of, and, you, for, it, in, that] | [“It’s like refusing ‘God’s kingdom come’.\n\nI often take positions opposite those of mainstream Christianity.\nBut not in this case.\n…”] |
-1 refers to all outliers and should typically be ignored. Next, let’s take a look at a frequent topic that were generated:
topic_model.get_topic(0) # Select the most frequent topic
[('game', 0.010318688564543007),
('team', 0.008992489388365084),
('games', 0.0071658097402482355),
('he', 0.006986923839656088),
('players', 0.00631255726099582),
('season', 0.006207025740053),
('hockey', 0.006108581738112714),
('play', 0.0057638598847672895),
('25', 0.005625421684874428),
('year', 0.005577343029862753)]
Access the predicted topics for the first 10 documents:
topic_model.topics_[:10]
[0, -1, 54, 29, 92, -1, -1, 0, 0, -1]
Visualize topics:
-1 refers to all outliers and should typically be ignored. Next, let’s take a look at a frequent topic that were generated:
topic_model.get_topic(0) # Select the most frequent topic
[('game', 0.010318688564543007),
('team', 0.008992489388365084),
('games', 0.0071658097402482355),
('he', 0.006986923839656088),
('players', 0.00631255726099582),
('season', 0.006207025740053),
('hockey', 0.006108581738112714),
('play', 0.0057638598847672895),
('25', 0.005625421684874428),
('year', 0.005577343029862753)]
Access the predicted topics for the first 10 documents:
topic_model.topics_[:10]
[0, -1, 54, 29, 92, -1, -1, 0, 0, -1]
Visualize topics:
topic_model.visualize_topics()
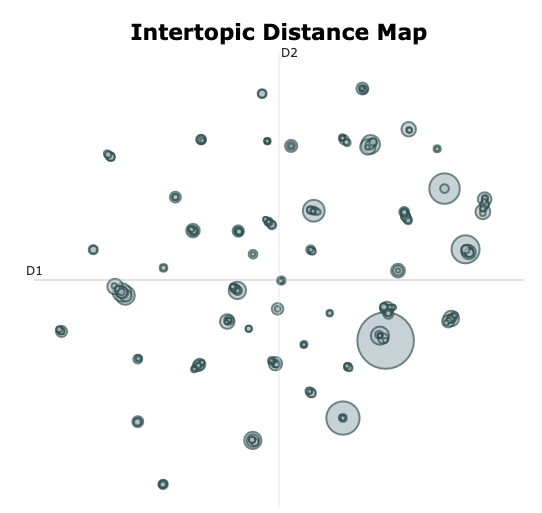
We can visualize the selected terms for a few topics by creating bar charts out of the c-TF-IDF scores for each topic representation. Insights can be gained from the relative c-TF-IDF scores between and within topics. Moreover, you can easily compare topic representations to each other.
fig = topic_model.visualize_barchart(top_n_topics=8)
fig.show()
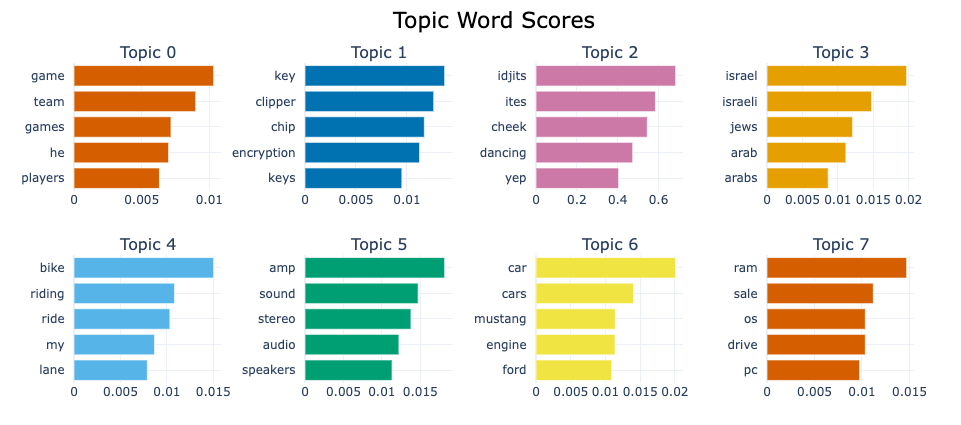